Data Privacy And Cybersecurity
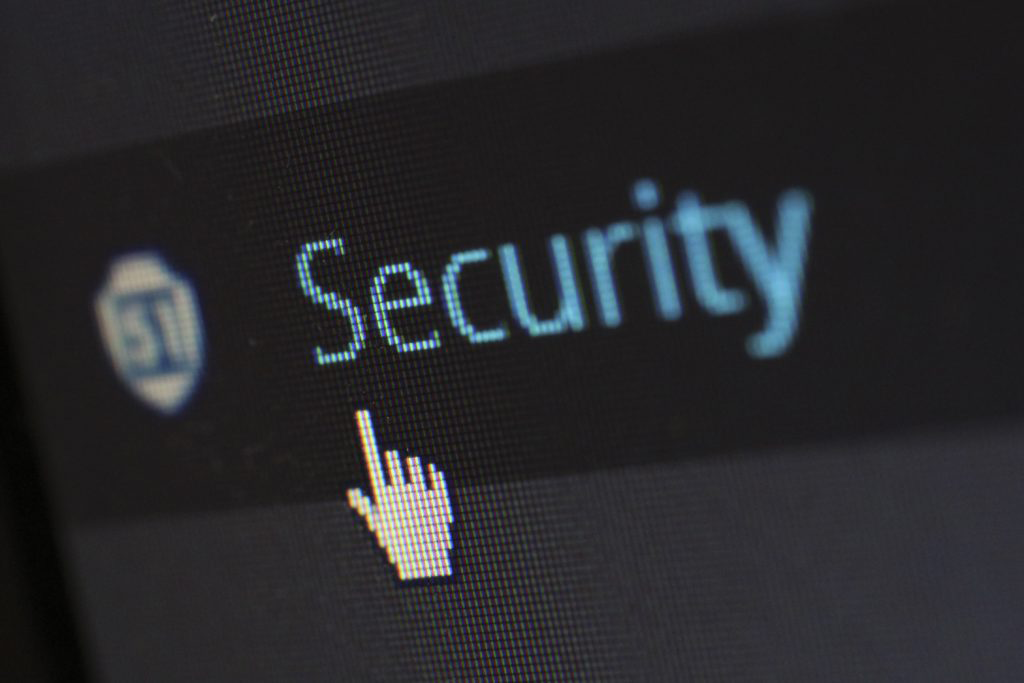
Understanding the Threats
The numerous points of entry into a connected and (fully) automated vehicle’s (CAV’s) computer system provide thieves and cyber terrorists multiple opportunities to take control of vehicles. For example, in 2010, more than 100 drivers in Austin, Texas found their cars disabled or the horns honking out of control, after an intruder hacking into an auto dealer’s computer system (Poulsen, 2010). Additionally, in 2015, two cybersecurity researchers hacked into a vehicle’s internal network and paralyzed it on a highway (Greenberg, 2016). While hackers like these can control non-AV through entry points like internal network systems, entertainment systems, hands-free cell-phone operations, and satellite radio, self-driving vehicles are even more vulnerable to attacks, because they have all of those entry points plus many more (Paisner, 2017). Cybersecurity is probably an overlooked area of research in the development of CAVs, even though many threats and vulnerabilities exist, and more are likely to emerge as the technology progresses to higher levels of automated mobility.
CAVs use what researchers call a cyber-physical system, with components in the “real” and virtual worlds. The safety stakes are as high as these systems are hard to protect. CAVs will be vulnerable to those that regularly disrupt computer networks, like data thieves of personal and financial information, spoofers who present incorrect information to a vehicle, and denial-of-service attacks that move from shutting down computers to shutting down cars. In addition, new threats unique to automated vehicles themselves emerge: hackers who would take control over or shut-down a vehicle, criminals who could ransom a vehicle or its passengers, and thieves who direct a self-driving car to relocate itself to the local chop shop, for example. Finally, there are security threats to the wide-ranging networks that will connect with AV: the financial networks that process tolls and parking payments, the roadway sensors, cameras and traffic signals, the electricity grid, and even our personal home networks (MCity, 2018).
Solutions
Automotive Industry
The automotive industry has addressed the issue of cybersecurity of AVs by creating a series of Automotive Cybersecurity Best Practices (2nd Auto-ISAC Cybersecurity Summit, 2018). The Automotive Information Sharing and Analysis Center issued the Automotive Best Practices, which guide how individual companies can implement the previously released “Enhance Automotive Cybersecurity” principle. The Automotive Best Practices covers organizational and technical aspects of vehicle cybersecurity, including governance, risk management, security by design, threat detection, incident response training, and collaboration with appropriate third parties. The Automotive Best Practices prompt participating members to enhance the security of self-driving vehicles by managing cybersecurity at the product level.
Federal Government
The federal government has also issued non-binding guidance to the motor vehicle industry for improving cybersecurity issues of AVs. The NHTSA first issued guidelines in October 2016. In an effort to reduce the probability of a successful cybersecurity attack, those cybersecurity best practices promote a layered approach to vehicle cybersecurity. For example, the NHTSA suggests that the automotive industry creates a culture of leadership where they can handle increasing cybersecurity challenges, mechanisms for information sharing, a documented process for responding to incidents, and more.The NHTSA has also warned that if the industry does not follow the guidelines, cybersecurity vulnerabilities will likely occur, and that such vulnerabilities may be considered safety defects compelling a recall. In September 2017, the NHTSA updated its guidelines from version 1.0 (NHTSA, 2016) to 2.0 (NHTSA, 2017). This updated version puts more emphasis on the importance of responding to incidents than the first version. The following is a summary of NHTSA guidelines (Paisner, 2017).
System Safety: Follow a robust design and validation process based on industry standards.
Operational Design Domain: Define and document the Operational Design Domain (ODD) for each self-driving vehicle available for use on public roadways. The ODD should include, at a minimum, roadway types, geographic area, speed range, environmental conditions, and other domain constraints.
Object and Event Detection and Response: Have a documented process for assessment, testing, and validating of the self-driving vehicle’s capabilities.
Fallback (Minimal Risk Condition): Have a documented process for transitioning to a minimal risk condition when a problem is encountered or the self-driving vehicle cannot operate safely. Fallback strategies should take into account that human drivers may be inattentive, under the influence of alcohol or other substances, drowsy, or otherwise impaired.
Validation Methods: Develop validation methods to appropriately mitigate the safety risks associated with their self-driving vehicle approach.
Human-Machine Interface: Consider and document a process for the assessment, testing, and validation of the vehicle’s HMI design.
Vehicle Cybersecurity: Follow a robust product development process that includes a systematic and ongoing safety risk assessment for each self-driving vehicle, the overall vehicle design into which it is being integrated, and when applicable, the broader transportation system. Document how your entity incorporates vehicle cybersecurity considerations into self-driving vehicles, including all actions, changes, design choices, analyses, and associated testing.
Crashworthiness: Consider incorporating information from the advanced sensing technologies needed for self-driving vehicle operation into new occupant protection systems that provide enhanced protection to occupants of all ages and sizes.
Post-Crash Self-Driving Vehicle Behavior: Consider methods of returning self-driving vehicles to a safe state immediately after being involved in a crash, such as shutting off the fuel pump, removing motive power, moving the vehicle to a safe position off the roadway, disengaging electrical power, and other actions that would assist the self-driving vehicles.
Data Recording: Establish a documented process for testing, validating, and collecting necessary data related to the occurrence of malfunctions, degradations, or failures in a way that can be used to establish the cause of any crash.
Consumer Education and Training: Develop, document, and maintain employee, dealer, distributor, and consumer education and training programs to address the anticipated differences in use and operation of self-driving vehicles from those of the conventional vehicles.
Federal, State, and Local Laws: Document how your entity intends to account for all applicable federal, state, and local laws in the design of their vehicles and self-driving vehicles.
Long-Term Data Privacy and Cybersecurity
Much work is still to be done on both data privacy and cybersecurity in the realm of AVs. As most developers of automated vehicle technology are working in pursuit of best-case scenarios, with a focus on optimizing vehicle performance, they may tend to overlook the specific and new issues that relate to automated vehicles. It’s crucial to create a thorough risk assessment approach and build long-term security solutions. So far, an initial step has been made by researchers who developed an assessment approach that can identify potential security breaches and the hackers behind them (MCity, 2018).